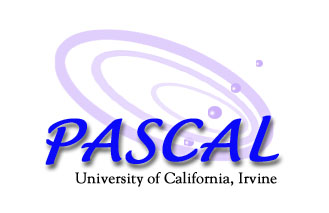
1. GPU Architecture
Rise of General-Purpose GPUs
The GPU was first developed to accelerate graphics processing, where there are a massive amount of instructions which have no dependencies with each other. With the emergence of the multi-core processors,the focus is now on parallel programming, where independent instructions are more favorable to parallelize. Seeing this, the GPU has now been extended to support general purpose instructions as well, by making it programmable on many cores (GPGPU: General-Purpose Programming on Graphics Processing Unit)
What We Are Doing
We model the GPGPU architecture, up to the hardware pipeline and memory hierarchy, consisted of local memory, global memory, constant memory, texture memory, shared memory, registers and buses, in order to increase performance and energy efficiency. The architectures are evaluated by a simulator implemented in C/C++, and virtually executing the instructions of the CUDA programming language
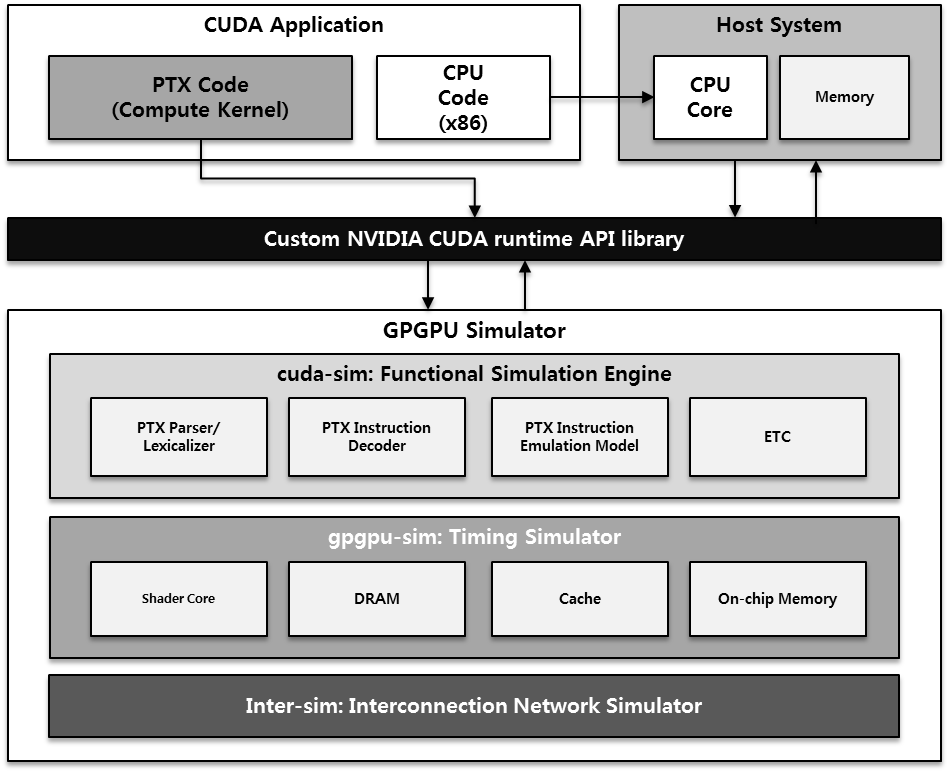
Publications:
- "Linebacker: Preserving Victim Cache Lines in Idle Register Files of GPUs " ISCA'19
- "FineReg: Fine-Grained Register File Management for Augmenting GPU Throughput" MICRO'18
- "WIR: Warp Instruction Reuse to Minimize Repeated Computations in GPUs" HPCA'18
2. Neural Processing Unit
What is a Neural Network?
Neural networks (NNs) are computing systems inspired by the biological neural networks that make up the human brain. NNs are based on nodes called neurons that model biological neurons in the brain. Like synapses, each connection can transmit signals to other neurons. Neurons that have received a signal can process the signal and send them to other connected neurons. At the connections between neurons are weights. They refer to the strength of the connection signal that adjusts at the learning stage. The neurons aggregate and form a layer. Each layer can have a different input processing method and can be classified according to the method (i.e., convolutional layer, a fully-connected layer). Due to its ability to reproduce and model nonlinear processes, NNs are used in many applications such as image recognition, speech recognition, and natural language processing
Why Are NPUs Necessary?
NNs have been mainly accessed using GPGPUs so far. However, since GPGPU is inherently hardware for general computation, it is hard to expect high performance for specific network algorithms. NNs are compute/memory-intensive algorithms and generally require a lot of computing power and high memory. For those reasons, both the academics and industry have been researching dedicated NPUs that provide more efficiency and acceleration
What We Are Doing
We model the NPU core. We analyze each NN application's operation characteristics and find a way to efficiently accelerate it by implementing the appropriate architecture, memory system, pipeline, and ISA. Also, we implement a simulator for NPU to analyze power, performance, and other bottlenecks
Publications:
- "Efficient Dilated-Winograd Convolutional Neural Networks" ICIP'19
3. Memory System
Applications mainly executed in datacenter (neural network, graph processing, database, etc.) require large memory space and have memory-intensive properties. However, the traditional processing-oriented computing paradigm has limitations in performance and performance when running modern applications. Because they are designed for computation, so processors have small on-chip memory space and large memory-access distance. Our research group conducts two fields to solve the performance and power problems of memory systems
DESIGNING A DOMAIN-SPECIFIC MEMORY SYSTEM
We study low-power, high-performance memory systems specialized for certain processors and applications, breaking away from the conventional general-purpose and formal memory hierarchy. We provide a memory solution that can solve the problem by identifying the unique characteristics of the applications that are mainly used or by finding the problem memory layer. Related research includes a neural-network-specific memory system and a heterogeneous memory system utilizing new memory
memory-centric computing platform
Memory-Centric Computing Platform refers to a system consisting of compute-nodes centered on memory, deviating from the existing processing-centric computing. In such a system, we do near-data processing. This is a study to minimize data movement between memory layers and accelerate operations by arranging the processing unit close to memory or storage. Related research includes processing in memory, database accelerating storage, and rack-scale pooled memory
Publications:
- "Check-In: In-Storage Checkpointing for Key-Value Store System Leveraging Flash-Based SSDs" ISCA'20
- "REACT: Scalable and High-Performance Regular Expression Pattern Matching Accelerator for In-Storage Processing" IEEE on Parallel and Distributed Systems 2020
4. Quantum Computer Architecture
What is Quantum Computing Architecture?
Quantum computers use the quantum bits(qubits) rather than the classical bits in classical computers. These qubits are in a complex interaction state known as quantum superposition and entanglement. Quantum computers use qubits to calculate quantum mechanics and solutions for various quantum algorithms
Quantum computing is currently a rising field in the computer architecture area and many sections are not yet standardized. Our lab focuses on quantum simulation and compiler optimization inside quantum computer architecture
What We Are Doing
Quantum Simulation Optimization
Real quantum computer machines are hard and costly to access for researchers. Thus, the quantum simulation is required in many areas of quantum research. However, due to the compute complexity differences of qubits on classical computers, it is required to have efficient resource management, such as memory bandwidth utilization and compute unit allocation
We focus on memory management since it is a major bottleneck in the state-of-the-art quantum simulators for larger sizes of qubits. Efficient quantum state indexing and data storing procedure is on research. We also exploit GPU thread level parallelism in simulation research to incorporate diverse promising topics
Quantum Compiler Optimization
Quantum compiler takes the role in translating high-level quantum language into quantum assembly form. It is essential to divide software algorithms with hardware instructions since machines only can handle very low level functionality. In this sense, a quantum compiler does qubit mapping, circuit scheduling, error control, and various other techniques. We research on qubit allocation and circuit generation steps to optimize for better hardware-friendly instruction level code
Collaboration
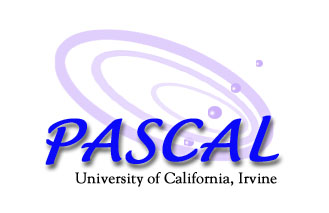
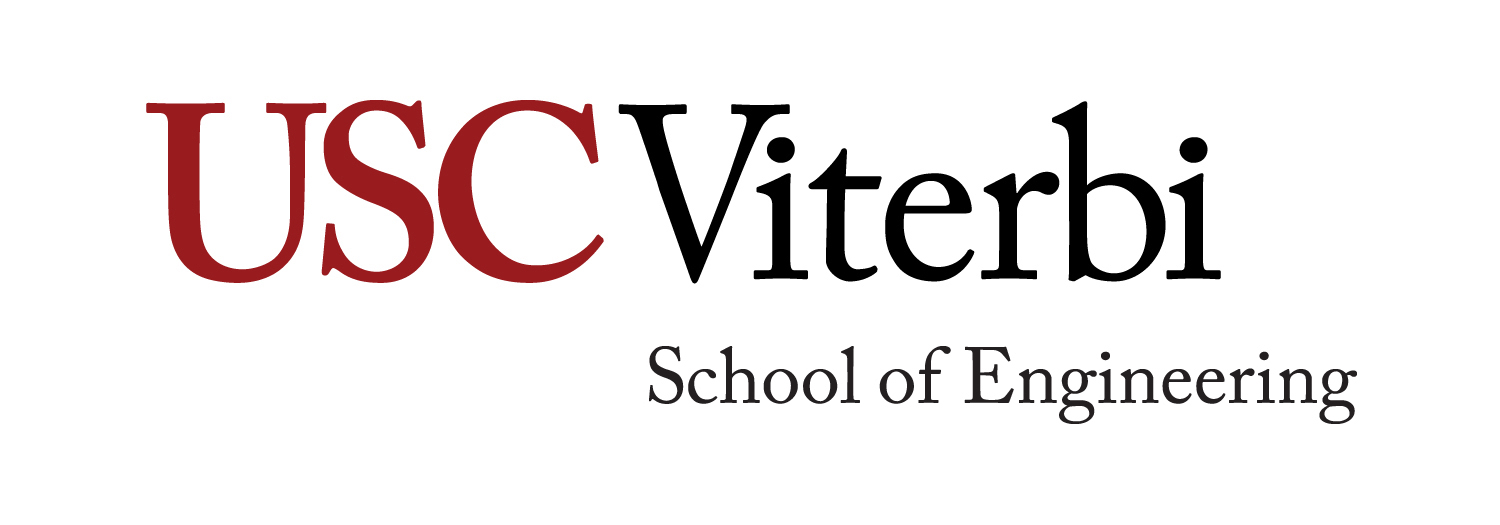
Super Computing In Pocket (SCIP) Research Group in the Ming Hsieh Department of Electrical Engineering, University of Southern California